Manipulation of deformable objects (DOs) offers unprecedented opportunities to resolve various real-world problems, such as binding and sealing tasks. Traditional technologies often focus on rigid-body manipulations and thus struggle with non-rigidity and high dexterity required for handling DOs. These challenges increase uncertainty and the burden of exploration in learning processes, necessitating not only sample-efficient learning strategies but also robust representation capabilities.
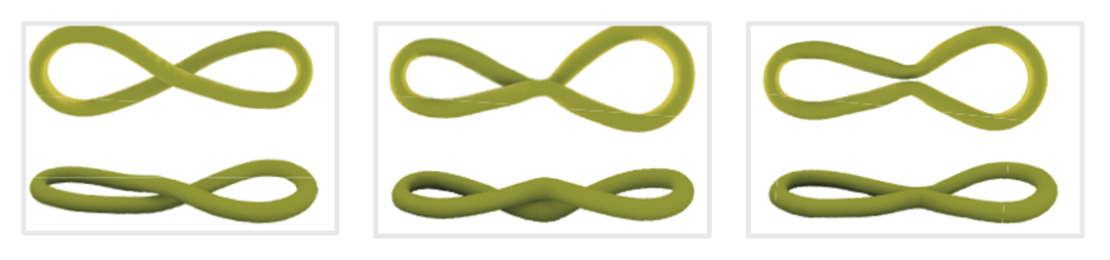
The research team led by Prof. Daehyung Park at KAIST has been pioneering solutions to state representation learning (SRL) problems in deformable object manipulation (DOM). This area is particularly challenging due to the infinite degree of freedom and nonlinear interaction dynamics that characterize the DOM processes. In DOM, dense yet partial observations, often due to self-occlusions, increase sampling complexity and uncertainty in policy learning.
To address these challenges, Prof. Park’s group introduced a novel approach termed implicit neural-representation (INR) learning for elastic DOMs (INR-DOM) (see Figure 2). This method is focused on learning consistent state representations of partially observable elastic objects by reconstructing their complete, implicit surface through a parameterized signed distance function (SDF), expressed by neural networks. SDFs are particularly advantageous as they describe object surfaces and provide spatial distance information crucial for physical interactions and manipulation planning.
Furthermore, the exploratory representation capabilities of INR-DOM are enhanced through reinforcement (RL), enabling RL algorithms to effectively learn exploitable representations and efficiently develop DOM policies.
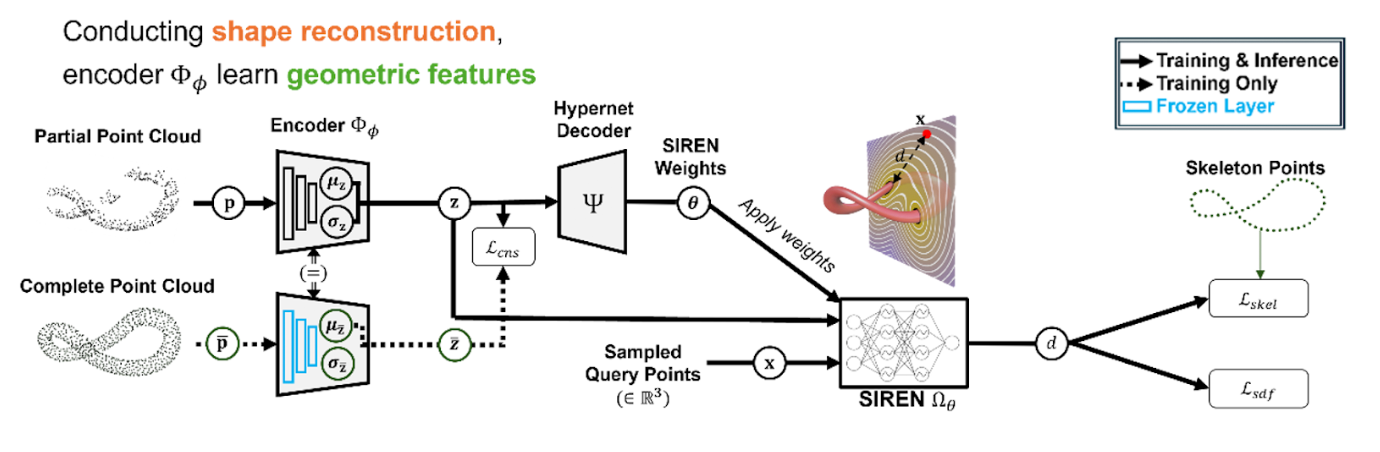
The efficacy of INR-DOM has been demonstrated across three simulated and real-world environments, involving tasks such as sealing, installation, and disentanglement. For example, using a Franka Emika Panda robot, INR-DOM successfully deformed and stretched rubber bands to meet various DOM goals. Notably, in a challenging disentanglement task, INR-DOM adeptly learned to distinguish twist directions, whereas ordinary vision-based approaches often fail due to visual ambiguities on the intersected bands. INR-DOM achieved a remarkable success rate of up to 91%.
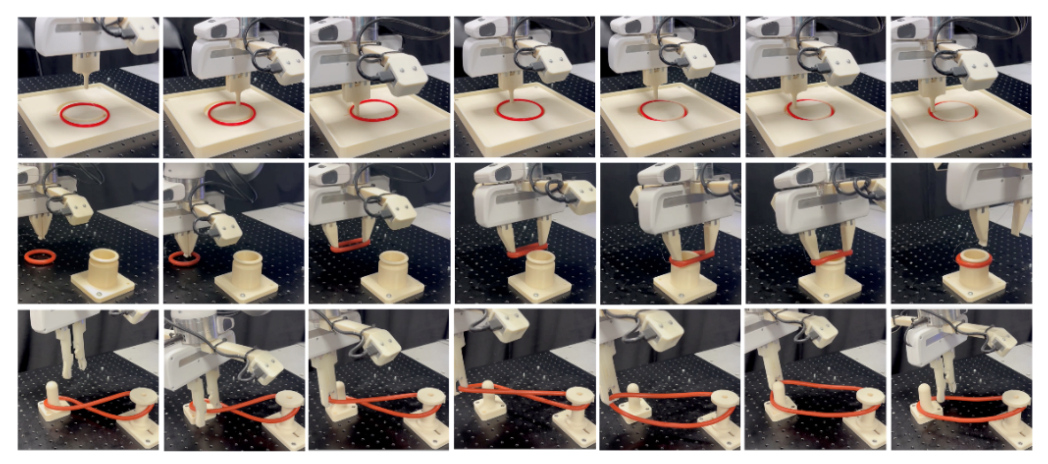
The paper detailing these findings is currently under peer review and available as an e-print at https://rirolab.kaist.ac.kr. For technical details, please refer to the eprint of our paper by J. Ha, M. Song, B. Park, and D. Park, titled “Implicit Neural-Representation Learning for Elastic Deformable-Object Manipulation.”
This work was supported in part by Samsung Electronics Co., Ltd, South Korea (No. IO220811-01961-01) and an Institute of Information & communications Technology Planning & Evaluation (IITP) grant funded by the Korean government (MSIT) (No. RS-2024-00509279 and No. RS-2022-II220311).
Prof. Daehyung Park School of Computing, KAIST
E-mail: daehyung@kaist.ac.kr
Homepage: https://rirolab.kaist.ac.kr