One of the key research directions in the deep generative model is to approximate data distribution using a modeled distribution. Recently, this model distribution has been developed as a diffusion model. The diffusion model approximates a distribution by minimizing the Fisher divergence, which compares the score function on distribution supports. This score function becomes the change function of data density, and diffusion models assume the change to be diffusion process over time. Diffusion models (a.k.a. score-based models) have been developed by Yang Song et al. at Stanford, and now they have become a de-facto standard for image synthesis in many real world applications.
AAILab at KAIST has developed various model structures and inference methods to synthesize realistic high-resolution images through diffusion models. First, Mr. Dongjun Kim at AAILab has suggested a new model structure to improve the score estimation through learning the nonlinear diffusion process and the discriminator to compensate the linear score estimation. The original diffusion model has suffered from the rigid linear diffusion process because of its virtue in closed-form solution for the forward-diffusion process. The contribution from Mr. Kim is the nonlinear forward diffusion process by merging the original diffusion model and the flow model. The flow model has a virtue of exact probability inference in the community of the deep generative model, so the merge enabled the closed-form solution as well as the nonlinear forward diffusion function. Additionally, Mr. Dongjun Kim suggested using a discriminator to compensate for the gap between the true data score and the approximated and pretrained score estimations. Mr. Kim showed that the discriminator can be integrated into the pre-trained score-estimation function by differentiating the discriminator by the parameters. This provides a fine-tuning method for existing pretrained diffusion models to better synthesize an image with the discriminator guidance.
Second, Mr. Dongjun Kim at AAILab discussed the problems of high resolution score estimations. The loss function of diffusion process can diverge if the diffusion has to handle extreme ends of high- and low-resolutions. Therefore, Mr. Kim suggested utilizing a soft-truncated weighting mechanism to reflect both extreme resolutions in regularized manners. This method enables the detailed synthesizing of high resolution images, while the synthesized images are still coherent with their structural aspects.
This work has been published as a paper in ICML 2022 and a paper in NeurIPS 2022. Also, AAILab expects another paper in ICML 2023 with the topics of discriminator guidance for diffusion models.
This research was supported by the program AI Technology Development for Commonsense Extraction, Reasoning, and Inference from Heterogeneous Data (IITP), funded by the Ministry of Science and ICT(2022-0-00077).
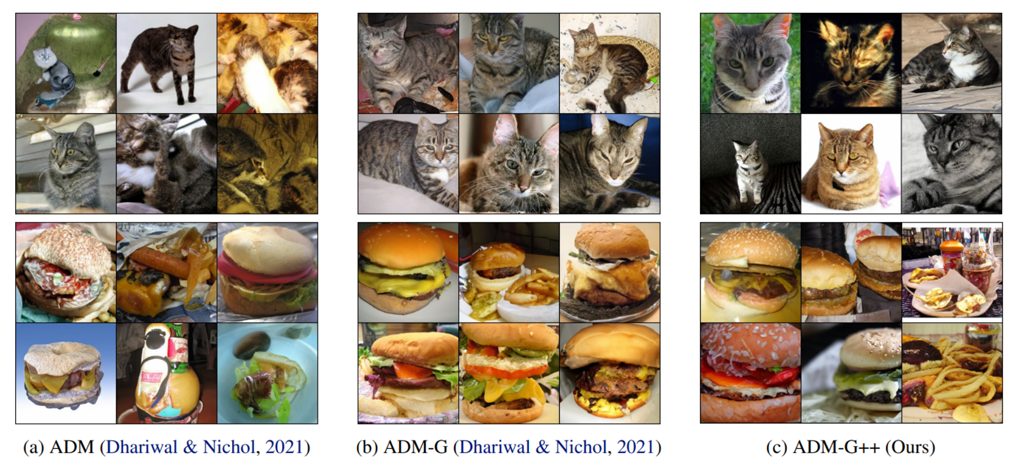
Mr. Dongjun Kim, Prof. IL-Chul Moon Department of Industrial and Systems Engineering, KAIST
E-mail: icmoon@kaist.ac.kr
Homepage: https://aai.kaist.ac.kr