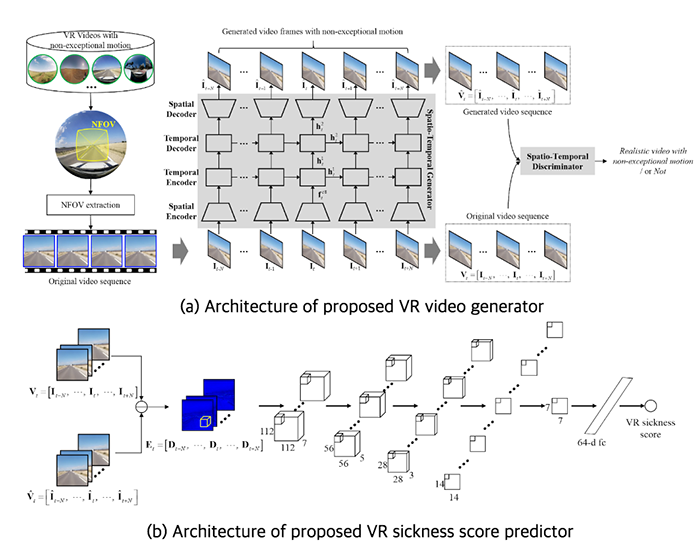
Virtual reality (VR) contents such as 360-degree video can provide realistic and immersive viewing experiences for viewers. With the growth of VR content services, concerns about viewing safety are increasing considerably. Many studies have reported various physical symptoms such as headache, difficulty focusing, and dizziness that could be caused by VR sickness. VR sickness is a bottleneck for the proliferation of the VR market.
To deal with this problem, a lot of time and effort have been devoted to the creation of viewing-safe VR contents. In addition, the viewing safety issue has been raised for user-generated VR contents as well. Therefore, it is essential to develop an objective VR sickness assessment (VRSA) that automatically predicts the degree of VR sickness. Most existing works have performed such evaluation of the level of VR sickness based on physiological measurements such as Electroencephalography (EEG) and galvanic skin response (GSR), and subjective questionnaires. These approaches were very cumbersome and labor-intensive due to extensive subjective assessment experiments. In addition, physiological measurement is generally vulnerable to various noises and subject movements, so that these methods can be inaccurate.
Dr. Hak Gu Kim and Prof. Yong Man Ro from the School of Electrical Engineering at KAIST (Image and Video sYstems Lab.) have developed a novel deep learning-based objective VR sickness assessment considering exceptional motion in VR content. The conflict between the visual sensor (eyes) and the vestibular sensor (ears) is one of the main causes of VR sickness (i.e., visual-vestibular conflict). Visual-vestibular conflict is largely caused by exceptional motion (e.g., acceleration and rapid turning) of content, since the physical motion of the viewer is relatively static. For example, when watching a 360-degree roller coaster video with an HMD, our visual sensor tells us that we are moving very fast, whereas our vestibular sensor tells us that we are not actually in motion. As a result, the discrepancy can lead to excessive VR sickness in the human motion perception system. Based on human motion perception, the researchers designed a new deep learning framework for VRSA, named VRSA Net, which consists of a VR video generator and VR sickness score predictor. In the training stage, the VR video generator is trained with normal videos with non-exceptional motion in order to reconstruct realistic normal video. As a result, the generator reconstructs VR videos well with non-exceptional motion. On the other hand, VR videos with exceptional motion, which can induce severe VR sickness, cannot be reconstructed well because the generator does not experience the videos with exceptional motion in the training stage. After obtaining the videos generated by the trained generator, the reconstruction error of the generated video is calculated. Because the VR video generator is trained with normal videos with non-exceptional motion, i.e., tolerable data for VR sickness in human motion perception, the reconstruction error could represent the discrepancy caused by exceptional motion. Finally, the VR sickness score predictor estimates the level of VR sickness by mapping the discrepancy information onto the corresponding subjective score. Experimental results show that VRSA Net had a strong correlation with human perception of VR sickness (PLCC: 0.885 and SROCC: 0.882). This research is the first attempt to automatically assess the overall degree of VR sickness based on VR content analysis using deep learning. The researchers hope this study can be used as a safety guideline when watching VR content or creating VR content in practice.
The results of this research, which was supported by the Institute for Information & Communications Technology Promotion (IITP), has been published in IEEE Trans. on Image Processing (Impact Factor: 5.072), a prominent international journal in the field of image processing, in April 2019 (volume: 28, issue: 4). From September, Dr. Hak Gu Kim, who is the first author of the paper, is starting his postdoctoral research at EPFL in Switzerland on deep learning in computer vision.
Dr. Hak Gu Kim, Prof. Yong Man Ro School of Electrical Engineering, KAIST
Homepage: http://ivylab.kaist.ac.kr/default
E-mail: ymro@kaist.ac.kr