Cameras have been used to capture visual information and have become essential sensors in many autonomous systems, such as self-driving vehicles, robots, drones, etc. However, conventional RGB cameras suffer from low dynamic range, motion blur, and low temporal resolution. Recently, event cameras, also known as neuromorphic cameras, were introduced. They are bio-inspired sensors that sense the changes of intensity at the time they occur and produce asynchronous events. Event cameras convey clear advantages such as very high dynamic range, no motion blur and high temporal resolution. However, since the outputs of event cameras are the sequences of asynchronous sparse events over time rather than actual intensity images, existing algorithms could not be directly applied. Although some methods have been proposed to reconstruct intensity images from event streams, the outputs are still low resolution (LR), noisy, and unrealistic. The low-quality outputs prevent broader applications of event cameras, where high spatial resolution (HR) is required as well as high temporal resolution, high dynamic range, and no motion blur.
To take advantage of event cameras in imaging under extreme conditions, the research team of Prof. Kuk-Jin Yoon in the Department of Mechanical Engineering has developed two novel Deep Neural Network (DNN)-based methods (supervised and unsupervised) that generate high-resolution (HR) intensity images from low-resolution event data while preserving the advantages of event cameras. In collaboration with a research team in GIST, a supervised-learning-based DNN framework and learning methodologies have been proposed (“Learning to Super Resolve Intensity Images from Events”, CVPR 2020). Meanwhile, an unsupervised adversarial learning framework that generates super-resolution images from event data has been proposed; this is a joint work with a research team from Imperial College London (“EventSR: From Asynchronous Events to Image Reconstruction, Restoration, and Super-Resolution via End-to-End Adversarial Learning”, CVPR 2020). The proposed technologies not only preserve the advantages of event camera but also make it possible to generate videos of very high framerates. These two methods are the first deep learning-based attempts to generate non-blurry, high-resolution, high-quality, and high-dynamic range images using sparse event streams of an event camera. Since these two methods (supervised and unsupervised) can be used differently depending on the availability of ground truth information, usability in real applications is increased.
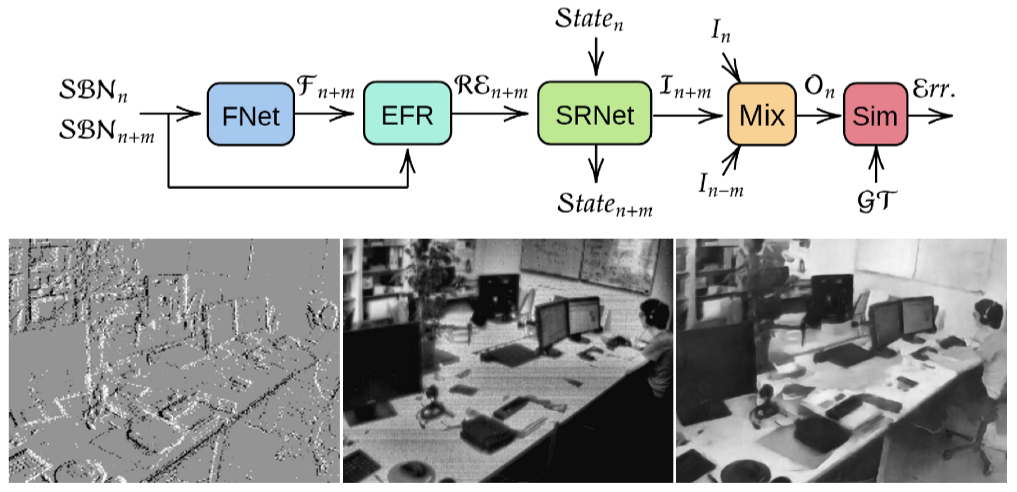
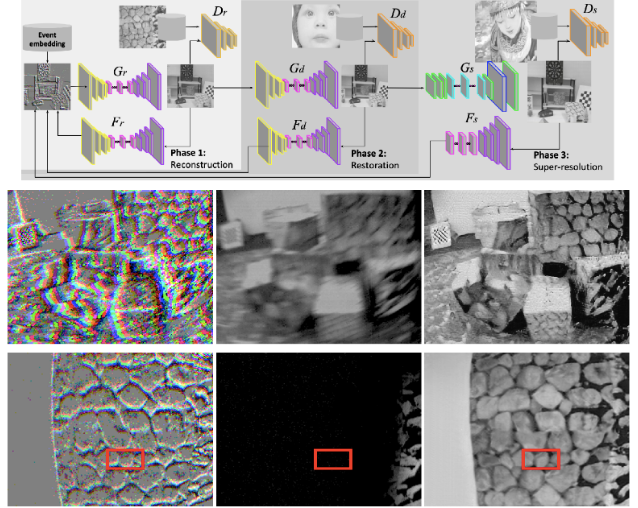

In June, these two studies were presented in the IEEE/CVF Conference on Computer Vision and Pattern Recognition (CVPR), a top-tier international conference on computer vision/machine learning. The results of these studies can be applied to many real-world applications and systems including autonomous driving vehicles, drones, and robots under extreme environments such as high-speed motion or huge illumination changes.
These works were supported by a National Research Foundation of Korea (NRF) grant (NRF-2018R1A2B3008640) and Next-Generation Information Computing Development Program through the NRF (NRF-2017M3C4A7069369) funded by the Ministry of Science and ICT, Korea.
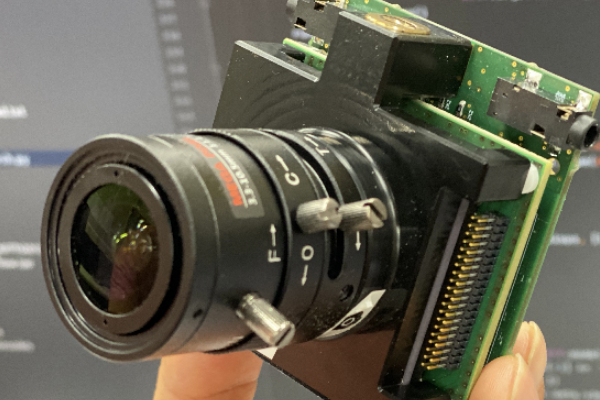
Prof. Kuk-Jin Yoon Dept. of Mechanical Engineering, KAIST
Homepage: http://vi.kaist.ac.kr
E-mail: kjyoon@kaist.ac.kr