There is great demand for developing a highly functional prosthetic hand in order to restore hand functionality to hand amputees. Over the years, significant research efforts have been devoted to enhancing the functionality of prosthetic hands through advancements in various fields, such as mechanisms, sensors, and control methods. However, effectively handling the high degrees-of-freedom (DOF) of a versatile prosthetic hand has remained a bottleneck.
The authors solved this issue by developing a novel perception system that can infer the grasp situation and intended grasp posture of the user in real-time. Proximity sensors placed at the palmar side of the prosthetic hand sense the distance between the sensor and the object while the absolute pose of the hand is tracked via an embedded pose tracking sensor (T265). Similar to a 3D scanner, this sensor system scans the surface of the target object as a 3D point cloud while the user extends the prosthetic hand toward the target object for grasping. Based on the acquired point cloud, the proposed system infers the user’s intended grasp posture and the size of the target object. A multi-layer perceptron classifier and a regression model were used for the grasp posture decision and object size estimation. The authors call this system Proximity Perception-based Grasping Intelligence (P2GI).
The P2GI system is applied to a prosthetic hand using the concept of shared autonomy. When the prosthetic hand user extends the prosthetic hand toward the object, the P2GI system perceives the object and plans the user’s intended grasp motion in the prosthetic hand. Then, when the user sends the grasp command via a simple single-channel EMG interface, the prosthetic hand grasps the object in the planned manner. As a result, the prosthetic hand user can utilize various grasp postures via a simple grasp command. The entire protocol was carried out in real-time without hesitation for scanning or inference. The delay from sensing to decision was less than 25ms.
The authors evaluated the P2GI system with 10 able-bodied subjects, and recorded 98.1% grasp posture decision accuracy and a 93.1% task success rate when grasping seven different objects while utilizing three different grasp postures. Notably, the performance is retained for unknown ADL (activities of daily living) objects; the subjects marked 97.8% grasp posture decision accuracy and a 95.7% grasp success rate for six unknown ADL objects. Since the proximity sensor used for the P2GI system is robust against environmental differences and object characteristics such as surface reflectivity, the proposed system shows robust performance in various unknown situations. With this advantage, the authors demonstrated the manipulation sequence for thirteen different ADL objects clustered in a cluttered environment.
By utilizing the proposed P2GI system, the authors expect that prosthetic hand users can intuitively operate aa dexterous prosthetic hand with higher convenience.
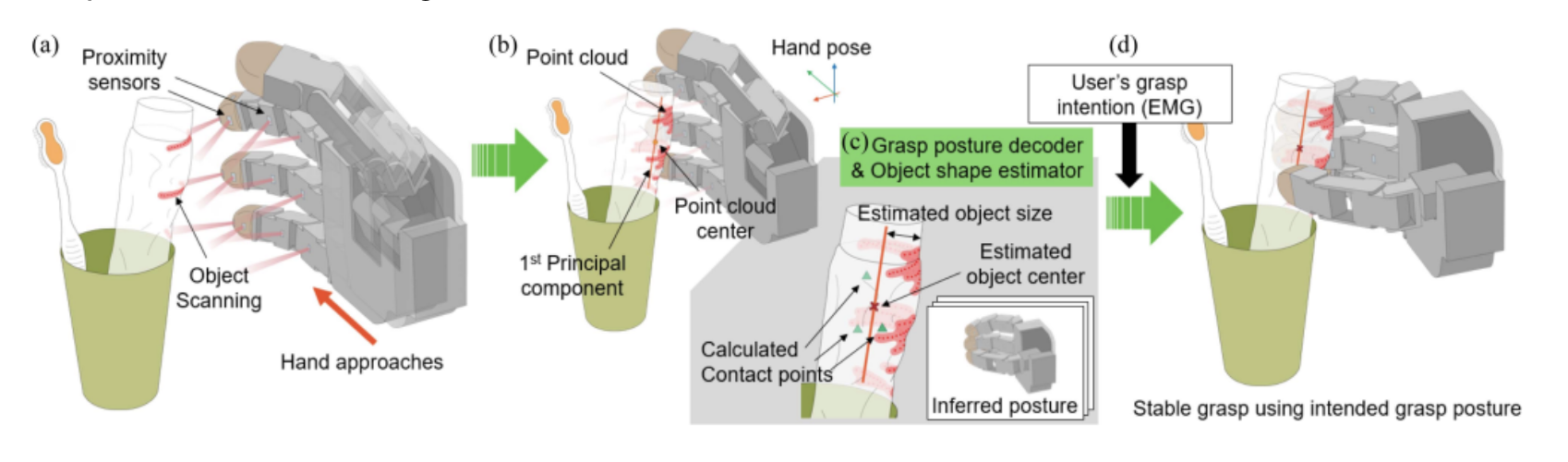
Prof. Hyung-Soon Park, Dr. Si-Hwan Heo Dept. of Mechanical Engineering, KAIST
E-mail: hyungsoon.park@gmail.com
Homepage: https://rehab.kaist.ac.kr